September 10, 2021
Finding a Way Through the Dark (Data)
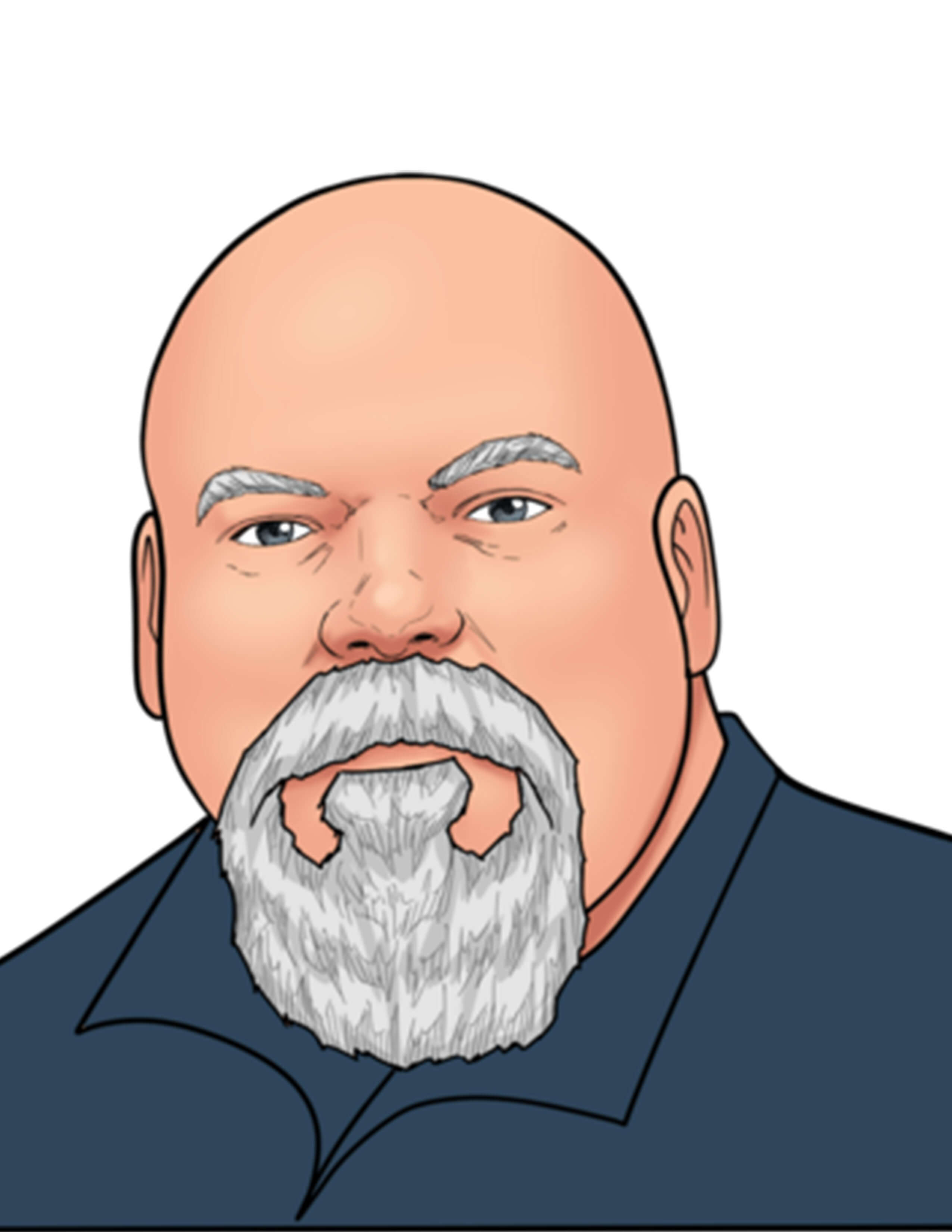
Dean Cornelison
It’s been recently said that data is the new oil. However, whilst gasoline is accessible and easily obtained from gas stations, the same cannot be said for data. Many organizations have tried to exploit data themselves, recognizing it as a valuable asset, however, entire industries have been created to assist companies to locate, gather, and assess data. Open source data sets are just one type of data that companies seek to collect to gain insight, identify security risks, and inform decision-making. The pitfalls and considerations of data privacy, compliance, and security all play a critical part in the data use ecosystem. This is particularly the case with open source data, which is publicly available data collected from the internet, which may include personal information and comes from a range of sources
The nature of open source data means that it is commonly located in open but not necessarily easily accessible platforms like the Deep or Dark web. This means that becoming proficient in this arena has become a recent subspecialty for a range of different sectors in society. Business, journalism, government, law enforcement, the military, and intelligence agencies are sectors that have all either adopted and/or have been the victim of data usage.
An end product of locating and aggregating data, assuming you have what you need, is information, insights, or intelligence. When you are missing data, whether it is internal, external or the data is something that you have failed to consider from other sources, this would be considered dark data. The lack of data or knowledge of how to view dark data has an interesting history.
In a famous news briefing, former U.S. Secretary of Defense Donald Rumsfeld said “there are known unknowns; that is to say we know there are some things we do not know. But there are also unknown unknowns—the ones we don’t know we don’t know.” Leaving aside the politics and history surrounding this statement, it actually hits the mark in how to consider a few classes of dark data.
In his book, Dark Data, author Dr. David J. Hand says “Dark data are concealed from us, and that very fact means we are at risk of misunderstanding, of drawing incorrect conclusions, and of making poor decisions. In short, our ignorance means we get things wrong.”
Dr. Hand describes several useful and interesting categories of dark data which are worth considering in your evaluation of data sources.
In Chapter 10, he lists the following:
DD-Type 1: Data We Know Are Missing
DD-Type 2: Data We Don’t Know Are Missing
DD-Type 3: Choosing Just Some Cases
DD-Type 4: Self-Selection
DD-Type 5: Missing What Matters
DD-Type 6: Data Which Might Have Been
DD-Type 7: Changes with Time
DD-Type 8: Definitions of Data
DD-Type 9: Summaries of Data
DD-Type 10: Measurement Error and Uncertainty
DD-Type 11: Feedback and Gaming
DD-Type 12: Information Asymmetry
DD-Type 13: Intentionally Darkened Data
DD-Type 14: Fabricated and Synthetic Data
DD-Type 15: Extrapolating beyond Your Data
In his book, Dr. Hand discusses how different ways of viewing or using data can greatly impact outcomes. Failure to seek out, gather and use all available data in a fraud investigation can have several serious adverse outcomes and is estimated to cost the US market billions of dollars every year.
From not understanding the true nature of the risk involved in underwriting a policy without the relevant background data or not properly determining the identity of a person under investigation, these outcomes all have downstream effects on determinations of coverage, liability, and damages. Legal, compliance, or regulatory concerns aside, the perception and impact of a poorly conducted investigation creates negative attitudes in the public if that case reaches the media. Public perception of business through social inflation is another factor to consider.
Additional exposures could involve misclassification of risk (either company involved or customers indicated data) which adversely affects premium calculations, improperly placing reserves with missing information. This results in adverse development and failure to obtain and investigate all of the actionable data involved in a loss.
Recognizing that obtaining and archiving open source and social media datasets early in the claims lifecycle, specifically at FNOL (First Notice Of Loss), prior attorney representation, or litigation are events that can make or break a case outcome.
Timeliness is especially important in cases where evidence, witness memories, or social media data may be lost. This confirms Dr. Hands’ dark data type “DD-Type 7: Changes with Time”. Obtaining data and evidence in a timely manner for future use can diminish exposure or even extinguish it.
On the other hand, unknown or unsought dark data (several of Dr. Hand’s categories apply here) such as background data on the nature of a business, its operations, drivers, training, and regulatory obligations can also have a significant impact.
What can the available data that we locate tell us about the behavior of the person or business we might be investigating? In his book, Psychology of Fraud, author Dr. Michael Skiba discusses the use of vulnerability assessment, controls, and monitoring. These are areas and concepts requiring extensive use of multiple data sets with a mindset of countering fraud.
The linchpin underlying all of these concepts is the need for and successful use of data. Crucial to that use is the location of internal and external data for creating processes and controls to implement a counter-fraud strategy. Dark data, whether internal or external, is a critical component in the success of any plan developed.
Dr. Skiba’s integration of counter-fraud processes and controls across business silos, which focuses on tying data to behavior to develop insights that can be leveraged, is insightful. The bridging of data and behavior is critical in finding open source and social media datasets that are actionable and material to an investigation.
Similarly, Dr. Hand discusses administrative and transactional data that results as a byproduct of a person’s digital activity, which he refers to as data exhaust. Utilizing keywords and behaviors in correlation with a person’s or business’ digital identity data set through open source and social media queries creates targeted searching with a specific eye toward behavior and identity.
In the present environment, where companies are dealing with unprecedented amounts of fraud and crime due to economic, social, cultural, and technological changes, the amount of data only increases daily. This creates the perfect storm of chaos in a culture that is facilitated by near real-time connected device usage and an overwhelming amount of data resulting from the presence of technology.
Utilizing dark data, especially considering data sets that may be missing yet crucial to evaluating the underwriting of risk, adjusting a claim, completing an investigation, or trying to solve a crime, can be vital to the success of an investigation. Leveraging open source and social media datasets is the way through the dark, and by leveraging this data you can limit your exposure to risk and further inform your investigations.